

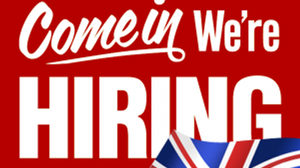

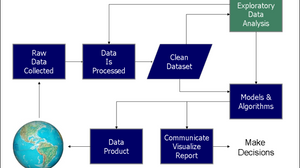
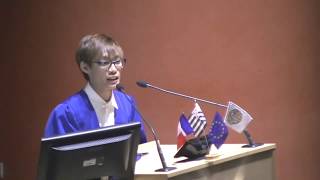
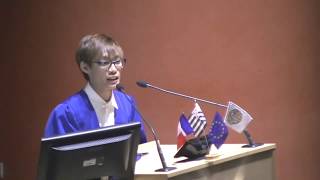
JUAN CARLOS MIGUEL CAMACHO
"...do to others what you would have them do to you..." (Matthew 7:12)
Recent Posts
Archive
Tags
Hi everyone (assuming someone is reading this)! It's been almost a month since I made my first blog entry. I promised myself that I'll post every two weeks but it proved to be harder than I thought. So maybe I can settle for a monthly post...well, only a tiny number of people have seen my blog anyway (and they were mostly my friends whom I forced to see my website) so I guess it doesn't matter lol.
This blog post is a technical or nerdy one, particularly on infectious disease surveillance and data science. Disease surveillance, specifically HIV/AIDS, is very close to my heart as it was my first job after I decided to shift my career from clinical nursing to public health (check my About page hehe); and is also the primary reason for my burning passion in epidemiology. Hence, I try to keep abreast with the rapid changes and advancement in the said field. (Wait, what is disease surveillance? Simply, it is the ongoing, regular collection of data regarding certain diseases with the purpose of establishing background information and/or impact of the disease, identify outbreaks, and evaluate health programmes to name a few).
I chose the title ‘Contagious Data Science’ because I would like to show the ‘contagiousness’ of data science and how it has spread in the field of epidemiology, particularly in infectious disease (ID) surveillance. I will mainly answer the question: ‘What data science can bring or has been bringing to ID surveillance?’ I will firstly have a brief overview of what data science is, and then move on with the SWOT (Strengths, Weaknesses, Opportunities and Threats) Analysis of data science in relation to ID surveillance or epidemiology at large by giving some examples along the way. Finally, I will discuss some of the ways forward for ID surveillance and epidemiology in light of data science.
To start with, what exactly is data science? I can't ask you individually but I’m sure that we’ll all give different answers and that's fine because data science is not a well-defined field yet. I thought that the best way to describe it is by presenting what data scientists do (image source: [1]):
They start with data collection, data processing, data cleaning, exploratory data analysis, creation of models, conduct analysis and communication of results [1]. What have you noticed? Strikingly, in terms of process alone, this is very similar with all the other fields! So the question now is: is data science only just a hype? I (and data scientists) would argue that it’s not, because one major difference lies in the kind of data they collect. Unlike surveillance for example, the raw data that data science collects come from the real-world that is generated in real-time. And this real-world data constitutes of what we all know as the ‘Big Data.’
This Big Data emerges because of the process of ‘datafication’ – a process of taking all aspects of our lives and turning them into data [1]. Aside from our online activities, even our offline behaviours are now datafied like the medicines we buy or the number of times we visited a gym. The goal of data science is to know these complex processes using the methods from various fields like statistics and computer science in order to understand the world better, and in turn, solve the world problems [1]. Having established the basic idea of data science, let us now move to the SWOT analysis of data science in relation to ID surveillance.
STRENGTHS: As I’ve discussed earlier, one of the components and strengths of data science is the Big Data which is often characterised by 3Vs [2]. The first V – high variety of data ranging from texts, tweets, emails, geo-based locations, images to videos. These are just few examples of the numerous sources of real-world data in which data science combines into a single data set that is ready for combined analysis. This now creates a very high volume data – the second V. Let’s take for example, Twitter. I am a Twitter user (follow me @migscam) and I am part of those who roughly produce 500 million tweets per day! Another example which everyone can relate, Google. There are around 4.5 billion searches happening in Google everyday! And these are just two of the many, many sources. This high volume allows us to have more number of observations or subjects, as well as more number of variables, that can be studied. And the last V – the high velocity data generation process wherein data are instantaneously compiled and can be analysed real-time or almost real-time.
WEAKNESSES: However, these strengths act as double-edged sword. One of weaknesses of Big Data is that the high volume and variety data we have now are very messy and coarse; so data cleaning and management can be more tedious. As what I’ve said earlier, it is not really a well-defined as a field yet especially in the academe. In the current discussion, there are opposing perspectives whether data science is a field on its own or is it just a hype-word for statistics. This debate is also being fuelled by media which make it sound like data science were just invented very recently and that Big Data only existed when Google came [1]. If we see it as a new field, these debates and media hype actually hamper the development of data science as a new academic subject.
OPPORTUNITIES: Let’s now move on to the opportunities that data science brings in ID surveillance which is the most exciting part of this blog entry! Given the emergence of new types and varieties of data, there is a massive opportunity for us (epidemiologists) to invent novel methods to analyse these kinds of data. This also meant that the methods in other fields like machine learning, which are not normally applied in epidemiology, are now being used in recent surveillance activities and studies. For example, the study done by Signorini et al. in 2011 [3] to track the swine flu pandemic using Twitter, they utilised SVR or Support Vector Regression to estimate the weekly influenza-like illness using tweets and compare it the actual cases from CDC. What did they find?
They found that the estimates of influenza-like illness using tweets (red solid line) was fairly accurate compared to the CDC reports (green dash line) with an average error of 0.28%. Isn't it cool?! Another example is a study done by Chan et al. in 2011 [4]. This time they used Google search queries to monitor dengue epidemics in five countries – let's take for example, Brazil:
The results showed that the model built by dengue-related queries in Google (red dotted line) was able to adequately estimate the true dengue activity based on the reports of the government and WHO (grey solid line). These information are very much promising for infectious disease outbreaks since real-time estimates provide a more timely results and earlier warnings for public health agencies, which allows for earlier prevention and response. These techniques could be a very valuable public health tool since reported data from government offices are usually available one to two weeks after the incident – which is still the biggest challenge of our current traditional surveillance systems.
Another opportunity is the generation of new forms of surveillance system in the form of web application hybrids [5]. A very good example is Health Map [http://www.healthmap.org/en/] which integrates various sources of online data to produce a global view of ongoing infectious disease threats. For example, this is a screenshot of what’s happening in the Philippines last week as shown in Health map:
We can see here that there is an ongoing gastroenteritis outbreak happening in Palawan province that was captured in an online news; and the best thing about this platform is that it is updated in real-time. These kind of ‘modern’ ways of doing surveillance can give us a view of health that is fundamentally different and more sensitive as they can capture data or cases that may not be captured in traditional surveillance. Lastly, the application of data science in disease surveillance can help in reducing public health costs as web-based approach analysis requires minimal resources [5].
THREATS: Despite these immense opportunities, data science also brings threats or challenges to ID surveillance. The first and biggest of them all, some data scientists argued that there is now no need to establish causation [6], which by the way is the very essence of our epidemiological practice. They argue that with high volume Big Data, we can now shift from causation to correlation (say what??). The second biggest threat is that some data scientists argues that the high volume Big Data may allow us to accept low-quality data which have lots of noises [6]. But again, this is unacceptable in our field as it poses validity challenges, which could make our results invalid and unreliable. Another issue is data ethics, such as data privacy, access and sharing [7]. Even though all our digital actions are datafied, not all information are for public. Or if they are public, access to big data is often limited and sometimes not accessible at all. And once you have access to it, data sharing is another issue. Finally, the lack of training and skills for epidemiologists in terms of the methods employed in data science such as machine learning, which I learned nothing about considering that I just recently finished my Master’s education (2017).
To this extent, what are the recommended actions for us, epidemiologists, given these opportunities and threats? Mooney et al. [2] suggested the following. Firstly, we should be involved in the design stages of data collection systems to improve the validity of administratively collected Big Data. This is very important especially in our current time when almost any data can be used for epidemiological analyses (as what the studies above have shown). Secondly, we should be able to increase our understanding of theories and subject matter because having high volume of sample will likely always lead to low p-value, but this does not imply clinical or population health importance. There would be a need to improve our skills to distinguish a highly precise finding and clinical/public health significance. Lastly, we need to expand our current academic programmes to acquire technological and computational skills that are not traditionally employed in epidemiology.
In conclusion and to answer the question I posed earlier as to what data science has been brining to ID surveillance, data science simply has been gradually modernising our surveillance systems. This has been evident with the immense opportunities data science (the Big Data in particular) has to offer. However, epidemiological training and practice should be able to adapt to the challenges posed by this new emerging field.
Key References:
[1] Schutt R. and O’Neil C. (2013). Doing data science : straight talk from the frontline. 1st ed. Sebastopol, CA: O’Reilly Media, Inc.
[2] Mooney S.J., Westreich D.J., and El-Sayed A.M. (2015). Epidemiology in the era of big data. Epidemiology, 26(3): 390–4.
[3] Signorini, A., Segre, A.M., and Polgreen, P.M. (2011). The use of Twitter to track levels of disease activity and public concern in the US during the influenza A H1N1 pandemic. PLoS ONE, 6(5).
[4] Chan, E.H., Sahai, V., Conrad, C. and Brownstein, J.S. (2011). Using Web Search Query Data to Monitor Dengue Epidemics: A New Model for Neglected Tropical Disease Surveillance. PLoS Neglected Tropical Diseases, 5(5)
[5] Brownstein, J.S., Freifeld, C.C., and Madoff, L.C. (2009). Digital disease detection—harnessing the Web for public health surveillance. New England Journal of Medicine, 360(21): 2153-2157.
[6] Cukier, K., and Mayer-Schoenberger, V. (2013). The rise of big data: How it's changing the way we think about the world. Foreign Aff., 92, 28.
[7] Salathé M., Bengtsson L., Bodnar T.J., Brewer D.D., Brownstein J.S., Buckee C., et al. (2012). Digital epidemiology. PLoS Comput Biol, 8(7).
Tags: